Predicting Neighborhood Socio-economic Condition in NYC

Digital CEQR
Predicting Neighborhood Socio-economic Condition in NYC
Introduction
Building a digital CEQR that predicts the socio-economic conditions in NYC.
City Environmental Quality Review (CEQR) is a process that needs to be executed should any urban project may have an impact on the city's environment. Currently, the majority of the environmental analysis work is done manually by the employees from different NYC government departments with specific chapters assigned to. Manual environmental analysis requires tons of work hours, resources and communication between various private and public entities. InCitu came up with a great idea of using predictive analytics to increase the efficiency and accuracy of CEQR analysis. CEQR consists of 24 chapters of different environmental impact analysis, we will focus on Chapter 5: Socio-economic Conditions.
Problem Statement
Current Issues
New York City's only tool to measure displacement risk is through the project-specific CEQR process which has limited capabilities to provide comprehensive guidance for decision makers. Moreover, the process neglects some of the most important factors when determining socio-economic change.
Research Objectives
In this analysis, we aim to build an analysis which eases the decision process for CEQR officials by providing a more macro, timely, and accurate prediction of neighborhood changes to study residential and commercial displacement better.
Research Questions
1.
How do we accurately predict the risk of gentrification for a neighborhood by taking into account race, income, and built environment related variables for 1, 5 and 10 years into the future?
​
2.
How do we predict economic and demographic trends for 1, 5 and 10 years into the future to better accommodate CEQR decisioning?
Data Collection
American Census Survey data, NYC open evictions data and NYC sales and rental data are the main data sources we used to build our predictive models.
Predictive Modeling
We implemented both supervised & unsupervised learning models to understand and predict the gentrification condition, eviction risk and sales & rental price of a certain neighborhood for current years and for 5 and 10 years into the future. These aspects reasonably reflect the socio-economic condition of a neighborhood in New York City.
Visualization
Spatial visualization is the main technic we use in our project to show the neighborhoods' socio-economic change. Our visualizations show social change parameters such as gentrification and eviction risk and economic change parameters including rental/housing price trends.
Our Approach
Results
Prediction of Neighborhood Gentrification Risk
Logistic Regression
Based on our results for the supervised learning model, we achieved an 82% accuracy and 87% recall for the prediction of gentrification. The final results of this elimination resulted in an increase of accuracy scores to 82% with recall being close to 87%. Based on the results of the models, we were able to infer that a decrease in evenness of income distribution, decrease in evenness of educational attainment, decrease in the racial composition, decrease in crowding within structures with 10 or more units and spatial lags for home value, rent and income distribution all play a major role in suggesting whether or not a tract has gentrified.
​
​
K-mean Clustering
Those factors all play a major role in suggesting whether or not a tract has gentrified:
Based on the clustering results, the two types of tracts that we got had major differences in the change in median income, educational attainment, the population living in poverty, unemployed population, minority race population composition, housing in structures with 10 or more units and change in mobile units. For tracts where incomes significantly rose within the 5 year period, it was also observed that people living in structures with 10 or more units declined, indicating a possibility of ongoing displacement within tracts that have gentrified.
​
Decrease in evenness of income distribution
Decrease in crowding within structures with 10 or more units
Decrease in evenness of educational attainment
Spatial lags for home value, rent and income distribution
Decrease in the racial composition
Web Tool
We developed a visualization tool to show the results of this project. By accessing the page of the tool it is possible to select the visualization showing the trends of each one of the five factors described above. Also, on the page, we added the conclusion of the analysis for the Red Hook area, which is an area of interest to the sponsors of this project. It is worth noting that this is a minimum viable product version of the proposed tool, we believe further versions of this tool could be developed incorporating the analysis for other regions, so the work could be generalized.
​
Conclusion
With the support of machine learning techniques, we are able to predict the risk of the gentrification of neighborhoods in New York City using the five year ACS demographics and social vulnerability index dataset. The finds of this research also suggest that change in income and ethnicity related factors play important roles in predicting neighborhood gentrification risk. Since the city agencies currently only use project-specific CEQR analysis but excluding macro demographic and economic factors, our results can potentially provide them with a better understanding of city-wide displacement risk during the decision making process.
The K-means Clustering results demonstrate that the declining population in structure with 10 units or more is also an important indicator of gentrification. This conclusion also supplies a remedy option for the current CEQR analysis approach where only low-income tenants living in 1-4 unit buildings are considered vulnerable to displacement while excluding those living in larger buildings.
Our Team
Project Sponsored by
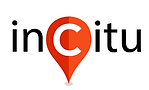